Collaborative Multilingual Continuous Sign Language Recognition: A Unified Framework
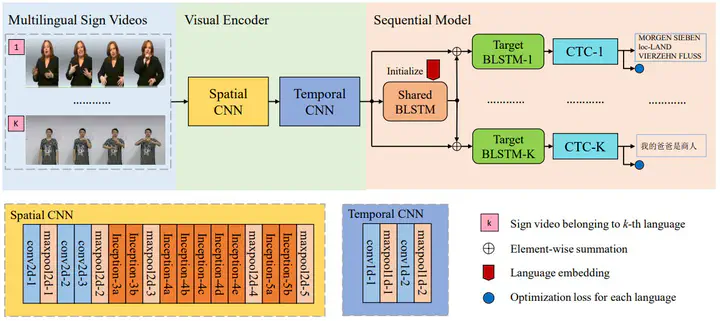
IEEE TMM 2022
Current continuous sign language recognition systems generally target on a single language. When it comes to the multilingual problem, existing solutions often build separate models based on the same network and then train them with their corresponding sign language corpora. Observing that different sign languages share some low-level visual patterns, we argue that it is beneficial to optimize the recognition model in a collaborative way. With this motivation, we propose the first unified framework for multilingual continuous sign language recognition. Our framework consists of a shared visual encoder for visual information encoding, multiple language-dependent sequential modules for long-range temporal dependency learning aimed at different languages, and a universal sequential module to learn the commonality of all languages. An additional language embedding is introduced to distinguish different languages within the shared temporal encoders. Further, we present a max-probability decoding method to obtain the alignment between sign videos and sign words for visual encoder refinement. We evaluate our approach on three continuous sign language recognition benchmarks, i.e., RWTH-PHOENIX-Weather, CSL and GSL-SD. The experimental results reveal that our method outperforms the individually trained recognition models. Our method also demonstrates better performance compared with state-of-the-art algorithms.